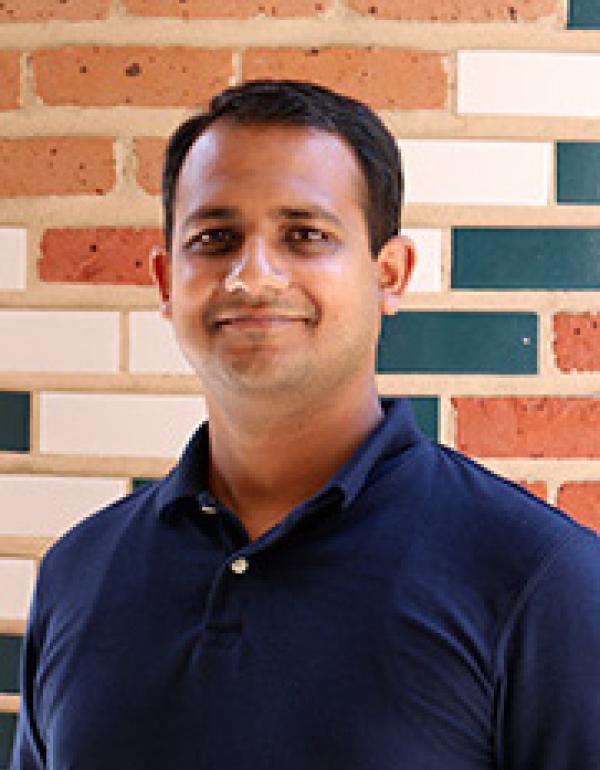
Research Interests: I am an applied mathematician who works on the the development of robust and mathematically sound numerical methods for solving partial differential equations (PDEs), with a special focus on conservation laws and fluid dynamics. I am particularly interested in the design of solvers that preserve key physical properties of the underlying PDE model, such as entropy stability, positivity and kinetic energy dynamics.
More recently, I have been working at the interface of conventional numerical analysis and machine learning. Along with my team, I focus on identifying computational bottlenecks in existing numerical algorithms and resolving them by the judicious integration of machine learning tools. I have used such techniques in several application areas, such as designing efficient shock capturing methods, designing deep learning-based surrogates to accelerate many query tasks, and solving inverse problems in the Bayesian framework.